Welcome to the clinical data market
- Amanda Berra
- May 22
- 10 min read
A primer to establish what's what before tackling the next-level question: What will the advent of synthetic data do to the clinical data market?
Having researched corporate innovation at health systems, I know system leaders have long been interested in figuring out ways to monetize clinical data. It makes sense: if systems could manage to sell or license de-identified data in their EHRs to life science or tech companies, that would be very helpful from a revenue diversification perspective. Especially whenever core inpatient economics are under siege.
But, it’s always been an easier-said-than-done proposition. And NOW, maybe there's also a new spoiler in the mix, in the form of 'synthetic data'. Synthetic data = AI-created, de-identified, no-patient-privacy-risk datasets that have the same statistical characteristics as actual clinical datasets.
I 100% guarantee you that at some point, YOU, yes you, are going to find yourself wondering whether and how AI-generated 'synthetic data' is poised to transform the still-nascent market for real-world clinical data sets.
How would this happen? Setting aside the possibility that you work at/have been approached about an investment in a synthetic data startup, you'll find yourself standing at this crossroads in one of these three ways, probably:
You work at a provider, payer, or tech/services organization with access to actual clinical data, at which executives/board have long been pushing to figure out more ways to monetize de-identified clinical data—and you'll be worrying about whether synthetic data is going to act as a spoiler.
You work at a life science or technology company that has been paying dearly for real clinical data—and you'll start wondering if synthetic data could be a viable—cheaper, less risk—alternative.
You work anywhere in healthcare and you'll be sitting in the audience at a panel going 'What in the world is happening??' as the panelists trade punches over this issue.
In this post, I’m going to work through PART 1 of the contextual knowledge we will need in order to have this discussion. Like this:
What is the clinical dataset market like currently (putting synthetic data aside for a moment)?
Why have systems struggled to realize the theoretical opportunity of monetizing clinical data?
What in the world is synthetic data—and, why would it potentially ruin
the clinical dataset market?
Cliffhanger alert! Keep a lookout for PART 2 of this post, which will address the main event: What is uptake of synthetic data like currently? What are the odds that it replaces market demand for real-world clinical data or, what effect is it likely to have on that market?
Preview: We think simply subbing in synthetic data will not be as cheap or easy as some seem to think. But put that aside for a second, because first things first. Let's take a tour of the clinical data market.
Background: What is the clinical data market? Where did it come from?
Short version: A few decades back, providers started storing data in EHRs. Over time, every provider organization (think health systems) has amassed a tremendous dataset that, if de-identified, can be a crucial resource for other stakeholders. E.g., accelerating life science work (e.g., by unlocking real-world evidence to discover new indications for existing drugs, or to assess efficacy) or to enable a wide range of healthcare tech/analytical solutions.
Why has it been ‘easier said than done’ to monetize clinical data?
Let’s start with technical know-how. To create data offerings that buyers value as highly as possible, health systems have to get through integrating and standardizing data from a patchwork of EHRs, imaging systems, and other sources. This is an uphill battle. Even once the data is aggregated, ensuring it is properly de-identified to meet HIPAA and GDPR (AKA, European HIPAA) requirements—without stripping away its analytical value—requires a ton of effort.
Beyond technical barriers, data monetization often calls for operational work—such as establishing new governance structures, negotiating data-sharing agreements, and working one’s way through the development of a lot of policies to address potential ethical, reputational, and compliance risks.
Last but definitely not least, as in any business line, clinical data doesn't productize/sell/optimize itself. A lot of work goes into packaging it, protecting it, connecting it to the market, etc. This work is pretty far afield from the core traditional mission and forte of health systems, i.e., healthcare delivery.
In short, while health systems MIGHT be sitting on a data goldmine, turning that asset into sustainable revenue is nothing like easy.
And that has been one reason that provider organizations have turned to other partners to work with this data, hoping that these partnerships will also yield various strategic results.
How provider partnerships with third-party aggregators have expanded the clinical data market
There are two major reasons why provider organizations have given their clinical data to others to sell: (1) Rational/strategic reasons, and (2) Byproducts of historical events/externalities from other goals.
(1) Rational/strategic reasons for providers to work with third-party aggregators
There are a lot of great reasons for providers to work with some kind of aggregator in the clinical data market, starting with the desire to increase the breadth and diversity of the data asset. As my colleague Eric Fontana explains, “It’s hard for any single health system to really provide the population and geographic diversity needed to extrapolate to a national market. Yes, some systems are multistate, but they then need longitudinal patient data and comprehensiveness of capture.”
Aggregators can offer a range of services that help connect the data to the market, making life easier for providers who usually aren’t geared up to get so far afield from their core focus (healthcare delivery).
Provider organizations also work with aggregators for privacy/risk mitigation reasons. As Eric puts it, “A solo organization marketing itself opens itself up to some potential problems that nobody wants. For example, if its data source gets hacked, all of a sudden that organization is going to be on the front of the WSJ, having incurred a breach while ‘selling their patients’ data.’” From that viewpoint, working with an aggregator is safer; the aggregator takes on the responsibility for security and safeguarding the data—along with the legal and reputational risk for any breaches.
(2) Byproducts of historical events
Providers might also give their data to aggregators to serve some other purpose--such as for the third party to analyze that data and return guidance or provide tech tools—leaving the aggregator in possession of a lot of clinical data that it can then repackage (with the appropriate data rights) and sell in the market for clinical data.
There have been at least two major catalyzing events like this in recent healthcare history.
Risk/VBC preparedness scramble: When getting up to speed on risk/population health became a big imperative in the mid-2000s, many providers hired outside partners to help do predictive analytics on patient populations.
Pooling data to help address Covid: In 2020, health systems began sharing data with one another in order to tackle Covid-related data challenges: Monitoring trends, identifying treatment levers, etc.
I think it's fair to say that providers have slowly become savvier about working with aggregators—while they may have signed permissive data licensing agreements in the beginning, allowing third parties to monetize the data on their own, it's become increasingly common at bigger systems for leaders to say "Hey wait a minute, this is a valuable resource—let's make sure we are looking out for our interests here". This led to the development of provider-facing aggregators ... see below.
Players in the clinical data market
As the clinical data market has matured, it has turned into a multi-party ecosystem. Rememebr that major player types generally also play in the CLAIMS data space, so without getting too complicated, here's a run down of GENERALLY who is who and what they do.
Providers and provider-DNA aggregators: Organizations monetizing member-organization or their own single-source clinical data, typically from EHRs.
Provider-DNA aggregators: Aggregate and harmonize de-identified clinical/EHR data from multiple provider organizations. Examples: Truveta, OMNY Health, ConcertAI, TriNetX
Single-source data sellers: Large health systems or academic medical centers selling their own de-identified data. Example: Mayo Clinic
Payer-DNA Aggregators: Organizations aggregating de-identified claims and, in some cases, clinical data, primarily from payer sources. Examples: Optum Life Sciences, Blue Health Intelligence
EHR Companies: Vendors with access to clinical data through their EHR platforms. Most do not openly license RWD, but some have developed networks or products for research and analytics. Examples:
Epic: Cosmos - large de-identified patient record database, not openly licensed but used for research and analytics
Cerner/Oracle: Oracle Health Real-World Data/Learning Network - offers researchers data from 100+ health systems
Flatiron Health: Oncology EHR, licenses de-identified data for research
Tech firms facilitating data marketplaces
Technology companies/platforms that build and operate data marketplaces, enabling exchange, discovery, or commercialization of clinical, imaging, genomic, or patient-contributed data.
Examples:
Avandra - medical imaging and clinical data
SCIKIQ - healthcare data marketplace
Tempus - AI-driven multimodal data for oncology and genomics
Helix - multi-institutional, longitudinal clinico-genomic registry
Citizen Health - patient-contributed data marketplace
Verily - harmonizes and curates EHR, genomics, imaging, labs, and more for research and analytics
5. Multi-source data platforms and aggregators
Differentiating among different players here is a challenge but here's a swing at it:
Platforms: Allow others to list data assets, facilitate transactions, provide linking/tokenization, and take a cut of licensing fees. Examples: HealthVerity, Datavant
Aggregators: Acquire, link, and package data from multiple sources (providers, payers, labs)--along with wraparound consulting services and tools IQVIA, Symphony/ICON, PurpleLab, Clarivate, LexisNexis Risk Solutions, Experian Health, Inovalon

Will the clinical data market always have so many players in it?
When we at Union look at the clinical data market, we see a market that is highly subject to network effects. Many buyers would love to work with one end-all-be-all, "foundational" data source they could use for all their purposes. And, as we know, healthcare organizations love to merge with/acquire each other. If/as any one data aggregator gets ahead of the rest in terms of depth/breadth of data organically—or, if/as the market consolidates, you could easily see the whole thing tipping toward a very small number of huge players, with only a few "niche" data sources left.
Just saying... watch this space.
Contract mechanics in clinical data market world
Still with me? Let's pause for one more moment on the key question of how money moves in the clinical data market: There are a lot of different, at least hypothetical, ways to make money on clinical data. Including:
Data-as-a-Service (DaaS): Offering curated, de-identified datasets to clients (such as life science companies, researchers, or payers) on a subscription or project basis.
Collaborative research networks: Pooling and selling access to data for clinical trial recruitment, observational studies, or multi-center research projects. Revenue may come from partnership fees, grants, or shared intellectual property.
Licensing: Granting third parties the legal right to use specific datasets under defined terms and for a fee. Can be exclusive (only one party has access) or non-exclusive (multiple parties can license the same data), and may be structured as a one-time payment, subscription, or pay-per-use.
Data marketplaces: Listing de-identified datasets on digital marketplaces where multiple buyers can purchase access, often with built-in compliance and transaction management.
Real-World Evidence (RWE) partnerships: Providing data to life sciences companies for post-market surveillance, drug safety monitoring, or real-world effectiveness studies. These partnerships often involve ongoing data feeds and analytics services.
Performance benchmarking and analytics services: Selling aggregated, anonymized data for use in benchmarking tools, dashboards, or analytics platforms that help clients compare performance or outcomes against industry peers.
Data-embedded services (including AI model training): Selling access to data to technology companies working to develop predictive models, clinical decision support tools, or other digital health products, AI/ML model training, or other capabilities/services.
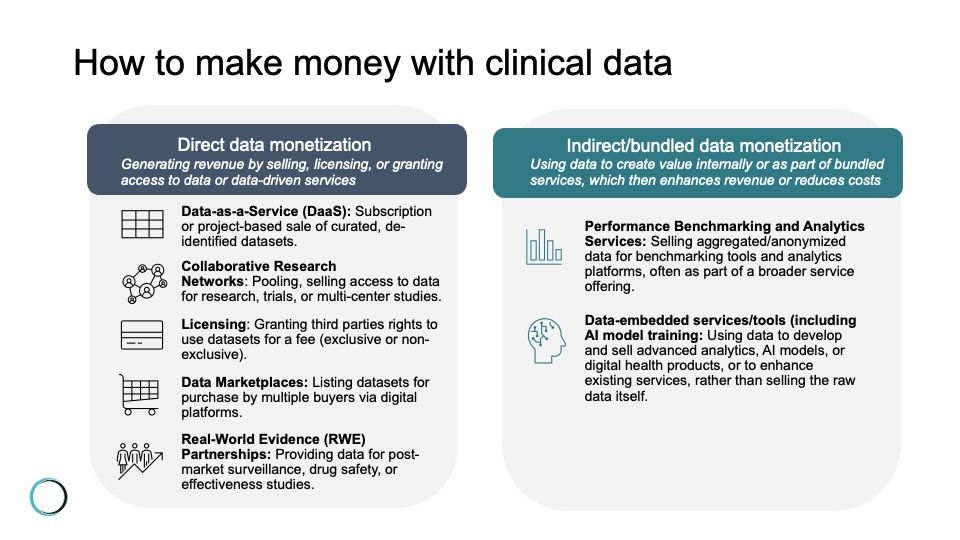
Pricing
Prices for access are highly variable. Two of the most critical dimensions that affect cost/revenue opportunity:
Completeness: Datasets that capture a comprehensive, longitudinal view of patient care—spanning multiple encounters and care settings—including as many data fields as possible—are more valuable. Completeness enables robust analytics, supports regulatory submissions, and reduces the risk of missing key clinical events.
Uniqueness: The more unique a dataset—whether by virtue of rare disease populations, specific geographies, or other angle for proprietary curation—the greater its value.
Other factors influencing value include data accuracy, integrity, and the degree of curation and harmonization. Regulatory constraints, context of data creation, and commercial purposes further shape the landscape, making some datasets more desirable for specific use cases or geographies.
For example: Let’s say what a buyer wants to do with a given clinical dataset is to understand utilization behavior. In that case, the claims data has to be ‘eligibility controlled’ (a.k.a "closed claims", not to be confused with the more commonly provider-focused use of the word "closed" which means paid claim) meaning that researchers have details to permit insight into an individual's insurance enrollment, which impacts utilization. Eligibility detail is usually only available from an insurer source.
OK so — what about synthetic data? What is it, at how is that playing in here?
For anyone thinking "surely there must be an easier way, especially in the age of AI" -- you're going to be a huge fan of the promise of synthetic data.
Synthetic data is artificially generated using advanced algorithms trained on real datasets. Its goal is to mirror the statistical properties and relationships of the original data without containing any actual patient information—which is how it potentially does an end-run around many hurdles, especially the ones that relate to privacy and compliance risk.
Synthetic data is typically created with advanced AI and machine learning algorithms. The tech under the hood includes generative adversarial networks (GANs), variational autoencoders (VAEs), and other generative models that learn from real datasets and then produce new, artificial data that statistically matches the original.
In theory, the end user could just swap this virtual data set in for the real thing—and support any analytical purpose that the real thing was being purchased for. (Again, we have some questions and thoughts here--to be explored in the next post.)
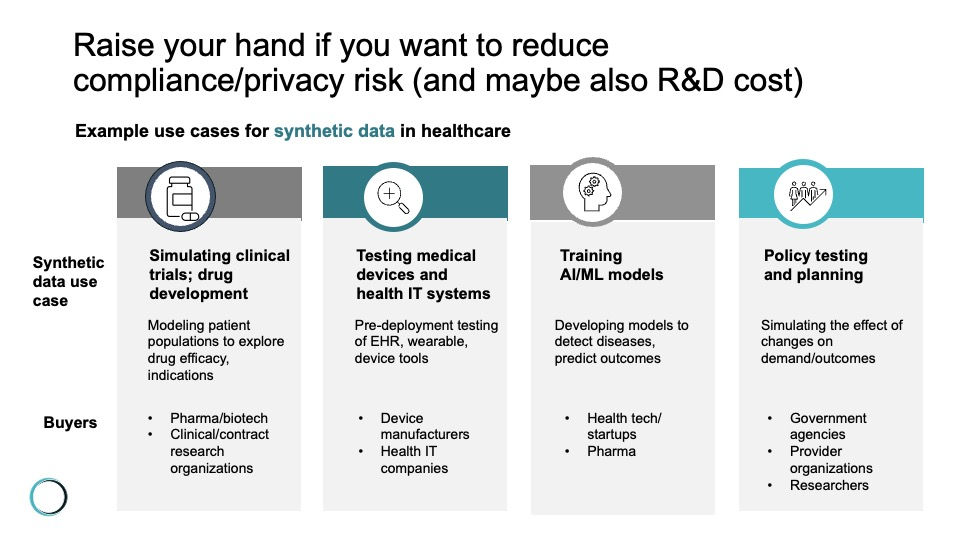
I see where this is going. So synthetic data could ruin the market for proprietary clinical data?
Yes—you're getting the picture. But just to spell it out: Synthetic data could undermine the proprietary dataset market by being cheaper and easier for the potential buyers of clinical datasets to use.
An “even better than the real thing” version of a clinical dataset would have virtues like bypassing regulatory and privacy constraints, enabling broader data sharing and collaboration, and presumably also being much cheaper. If clinical datasets get commoditized in this way—if the market doesn’t perceive a unique value proposition of proprietary datasets and synthetic data becomes “good enough” for most research and AI training purposes—well, again IN THEORY, that would ruin the market for real-world proprietary dataset sellers.
We’ve arrived at the cliff: Tune in for the next post to learn how synthetic data is affecting the market for clinical data
Hopefully you've enjoyed today's tour of the clinical data market. Including at least a brief intro of our dark horse candidate: Synthetic data.
But asking the question ‘what will synthetic data do to the market for clinical data’ opens the door into a whole new conversation—which I will post next.
Here's a preview of our thoughts on this question--we question the idea that synthetic data will be as cheap/as easy to implement as a replacement for real clinical data as synthetic data proponents tend to argue. (Yes, there is such a thing as a synthetic data proponent, you will know when you meet one.) If you have thoughts on what role synthetic data will play in the future market for clinical data, now is the time to reach out!
And don’t forget to subscribe to the blog so you receive an alert (scroll down to enter your email address).
Comments